Table of Contents
The healthcare industry is transforming, and artificial intelligence (AI) is leading the way. AI is streamlining processes, improving accuracy, and enhancing patient care. In ophthalmic diagnostics, AI revolutionizes how eye diseases are detected, diagnosed, and treated.
According to a report from Keragon, over 10% of healthcare professionals in the United States already use AI, and nearly 50% plan to implement it shortly. This rapid adoption highlights the growing trust in AI-driven diagnostic tools and their potential to enhance clinical efficiency.
Eye diseases such as diabetic retinopathy, glaucoma, and age-related macular degeneration (AMD) require early detection for effective treatment. AI-driven systems are invaluable in identifying these conditions sooner, often outperforming traditional methods.
This guide will examine how AI is changing eye care. It will cover AI’s role in eye health and the latest trends and challenges in using AI. We will discuss new diagnostic tools and their benefits. We will also share real-world case studies showing how AI helps eye care.
The Role of AI in Modern Ophthalmology
AI is revolutionizing ophthalmology management by enhancing diagnostic precision. This minimizes the workload for specialists and delivers faster, more accurate results.
AI-powered diagnostic tools leverage deep learning models to analyze retinal scans, optical coherence tomography (OCT) images, and fundus photographs with unprecedented efficiency. These tools can detect minute abnormalities the human eye may overlook, ensuring earlier and more reliable diagnoses.
Additionally, AI enhances workflow automation, allowing ophthalmologists to focus on patient care rather than administrative burdens. This leads to a more streamlined and effective diagnostic process.
How AI Is Changing Ophthalmic Diagnostics
The integration of AI into ophthalmology is revolutionizing diagnostic accuracy and efficiency. As AI-driven technologies evolve, their impact on early detection and treatment of eye diseases becomes increasingly profound.
AI-Powered Pattern Recognition in Ophthalmology
AI algorithms detect intricate patterns that may be imperceptible to the human eye. This allows for the identification of early indicators of diseases such as:
- Diabetic retinopathy
- Glaucoma
- Macular degeneration
By leveraging deep learning, AI can analyze vast datasets and recognize even the most subtle retinal abnormalities, facilitating timely and accurate diagnoses.
AI-Driven Automated Screening in Ophthalmology
Automated screening solutions use AI imaging tools to help ophthalmologists. They can quickly assess thousands of retinal scans, allowing specialists to focus on high-risk cases more efficiently. These systems improve patient throughput and allow ophthalmologists to focus on complex cases requiring human expertise.
AI-Powered Remote Diagnostics in Ophthalmology
AI systems enable remote diagnostics. It offers a breakthrough in delivering eye care to underserved and rural regions.
Teleophthalmology powered by AI allows patients to receive screenings and preliminary diagnoses without visiting a specialist in person. AI-assisted platforms can analyze images captured through mobile-based fundus cameras and other portable devices. It ensures that more individuals can access timely and accurate eye disease detection, regardless of geographical limitations.
The Technology Behind AI in Ophthalmology
AI-driven technology is reshaping ophthalmology by enhancing diagnostic precision and automating complex analyses. As these innovations continue to advance, they are setting new standards for efficiency and accuracy. It ultimately improves patient outcomes and clinical workflows.
Key AI Technologies in Ophthalmology
AI technologies are crucial in advancing diagnostic accuracy and efficiency in ophthalmology. These innovations empower ophthalmologists with data-driven insights, enhancing their ability to detect and treat eye diseases more precisely.
Machine Learning & Deep Learning
AI models trained on large datasets improve accuracy over time. These models learn from vast amounts of retinal imaging data and can detect subtle abnormalities that human observers might miss.
They also adapt and improve by seeing new patient cases. This helps them better distinguish normal from abnormal retinal structures.
AI-based deep learning systems provide automated diagnostics. These systems assist ophthalmologists in reducing diagnostic variability and enhancing decision-making speed.
Image Recognition Algorithms
AI detects disease markers in retinal images with high accuracy. These algorithms use deep convolutional neural networks (CNNs) to look at features in retinal scans. They can find signs of conditions like diabetic retinopathy, glaucoma, and macular degeneration.
CNNs enable AI to recognize microaneurysms, hemorrhages, and other pathological changes with a precision that rivals human experts. AI-powered image recognition streamlines the diagnostic workflow by flagging high-risk cases for immediate attention. This ensures early intervention and reduces vision loss rates.
Natural Language Processing (NLP)
Assists in analyzing medical reports and research studies for better insights. NLP-powered AI tools can read and understand many ophthalmology literature, patient records, and clinical notes. They extract important data that helps healthcare professionals make better decisions.
These AI-driven systems can:
- Identify trends in patient symptoms
- Suggest relevant clinical studies
- Summarize complex findings
This makes it easier for ophthalmologists to stay updated with the latest advancements.
NLP helps improve patient interaction. It analyzes spoken or written descriptions of symptoms, making preliminary assessments more accurate. It also enables better communication between doctors and patients. This technology allows for faster and more efficient information retrieval, reducing administrative burden and streamlining diagnostic and treatment processes.
Predictive Analytics
AI-driven predictive models analyze patient history, lifestyle factors, and genetic data to assess the risk of developing specific eye conditions. These models utilize vast datasets and machine learning algorithms to identify patient health record patterns. They recognize potential disease indicators before symptoms manifest.
AI-powered predictive analytics help eye doctors identify patients at high risk for conditions like diabetic retinopathy, glaucoma, and age-related macular degeneration and provide personalized treatment recommendations.
This allows ophthalmologists to implement preventive measures, such as:
- Lifestyle modifications
- Targeted screenings
- Early interventions
These preventive measures reduce the likelihood of severe disease progression and improve long-term patient outcomes.
AI-Assisted Optical Coherence Tomography (OCT) Analysis
AI enhances OCT imaging by identifying intricate retinal layer changes that may indicate early disease onset. AI helps speed up and improve diagnosing conditions like diabetic macular edema and glaucoma. It does this by automating the segmentation and analysis of OCT scans.
AI-powered OCT analysis can detect minute structural changes in the retina, even before symptoms manifest. This allows for earlier interventions and better patient outcomes.
Additionally, AI-driven OCT systems reduce human error and variability in diagnosis, ensuring consistent evaluations across different practitioners. These advancements improve the efficiency and reliability of retinal disease detection. They ultimately lead to enhanced treatment strategies and personalized patient care.
Common Applications of AI in Ophthalmology
AI is reshaping modern ophthalmology by automating processes and improving diagnostic capabilities. As AI-powered systems evolve, they significantly enhance efficiency and accuracy in clinical decision-making.
AI is enhancing multiple aspects of ophthalmic diagnostics, such as:
- Revolutionizing early disease detection
- Improving diagnostic accuracy
- Optimizing patient management
AI-driven tools do the following:
- Enable more efficient workflows
- Empower ophthalmologists with real-time insights to provide better patient care.
AI is enhancing multiple aspects of ophthalmic diagnostics, including the following.
Automated Detection of Retinal Diseases
AI detects diabetic retinopathy, AMD, and glaucoma through high-resolution imaging. These AI-driven tools analyze fundus photographs and OCT scans. They identify minute changes in the retina that could indicate the early stages of disease progression.
Machine learning algorithms help AI improve its accuracy. They learn from large datasets. This allows AI to tell the difference between normal and abnormal retinal structures precisely. Early detection through AI-assisted screening reduces the risk of vision loss by facilitating timely medical interventions.
Screening for Cataracts
AI tools analyze lens opacity and predict the severity of cataracts. Advanced AI-based imaging techniques assess clouding in the eye’s lens. This detects early signs of cataract formation before symptoms become pronounced.
AI models can quantify the degree of lens opacity. They help ophthalmologists determine the appropriate timing for surgical intervention.
These automated screening solutions provide consistent evaluations, reducing the subjectivity associated with manual cataract grading and improving patient care outcomes.
Corneal Analysis
AI-powered systems assess corneal abnormalities for early disease detection. AI-driven topography and tomography tools allow for highly detailed mapping of the cornea. This enables early identification of conditions such as:
- Keratoconus
- Corneal dystrophies
- Post-surgical complications
These AI systems analyze corneal thickness, curvature, and shape exceptionally. They assist ophthalmologists in better diagnosing and treating corneal diseases.
Additionally, AI-enhanced imaging can improve the outcomes of refractive surgeries like LASIK by providing highly accurate preoperative assessments.
Telemedicine Integration
AI enables remote diagnosis, reducing the need for in-person visits. AI-assisted teleophthalmology platforms analyze retinal images and patient data in real-time. They provide automated assessments that help ophthalmologists offer remote consultations with greater accuracy.
These technologies are particularly valuable in rural and underserved areas. They ensure patients receive timely diagnoses and referrals without requiring travel to specialized centers.
Combining AI-powered diagnostic tools and telemedicine bridges gaps in eye care accessibility. Therefore, it improves early detection and treatment outcomes for patients worldwide.
Implementing AI in Ophthalmic Practices
The adoption of AI in ophthalmology is transforming clinical workflows and patient care. Integrating AI-powered solutions into ophthalmic practices is essential for maximizing their benefits and ensuring smooth implementation.
Steps to Integrate AI into Eye Care Clinics
Integrating AI into ophthalmic practices requires a strategic approach to ensuring efficiency and compliance. By following structured implementation steps, clinics can maximize AI’s potential to enhance diagnostics and patient care.
1. Assess AI Readiness
Identify clinic needs and select appropriate AI-powered diagnostic tools. Conduct an in-depth analysis of the clinic’s current diagnostic capabilities, identifying gaps that AI can address. This includes evaluating existing diagnostic methods’ accuracy, efficiency, and limitations to determine where AI integration can provide the most value.
Consideration should also be given to the clinic’s:
- Patient volume
- Workflow efficiency
- Staff expertise
This ensures a well-informed AI adoption strategy. To provide a seamless transition, evaluate available AI solutions based on:
- Compatibility with existing equipment
- Ease of integration
- Cost-effectiveness
This ensures a seamless transition. Conduct pilot testing of AI technologies to assess their real-world effectiveness before full-scale implementation, ensuring minimal disruption to patient care.
2. Train Staff
Ophthalmologists and technicians require training to interpret AI-generated reports accurately. Comprehensive training programs should be implemented to familiarize healthcare providers with AI-driven diagnostic systems.
Hands-on workshops and continuous education sessions will help medical professionals build confidence in using AI tools and integrating them into their clinical workflows.
3. Adopt AI-Driven Workflows
AI can streamline scheduling, patient triage, and treatment planning. AI-powered administrative systems can automate appointment scheduling, reducing wait times and optimizing patient flow.
Clinics can better prioritize urgent cases by leveraging AI-driven triage, ensuring that patients with severe conditions receive immediate attention.
Additionally, AI can assist in treatment planning by analyzing patient data and suggesting tailored interventions.
4. Ensure Regulatory Compliance
AI adoption must align with healthcare regulations and data security policies. Implementing AI in ophthalmology requires adherence to strict patient data protection laws, such as:
- HIPAA in the United States
- GDPR in Europe
Ensuring AI systems comply with these regulations involves:
- Encrypting patient information
- Maintaining secure data storage
- Updating software to meet evolving standards
Clinics must also ensure transparency in AI-driven decision-making to build trust among patients and healthcare professionals.
Case Studies: Successful AI Integration in Eye Care
Several hospitals and eye care centers have implemented AI to improve patient care. These institutions have leveraged AI’s advanced imaging and diagnostic capabilities to enhance early detection rates and streamline treatment workflows.
IDx-DR AI System
The IDx-DR AI System is an FDA-approved device. It uses artificial intelligence to find more than mild diabetic retinopathy (mtmDR) in adults with diabetes. The system captures detailed retinal images using advanced imaging techniques such as optical coherence tomography (OCT) and fundus photography. Sophisticated AI algorithms then analyze them to identify and classify diabetic retinopathy.
This non-invasive, rapid, and reliable diagnostic tool assists healthcare professionals in making timely and informed clinical decisions, thereby improving patient outcomes.
Aravind Eye Hospital & AI-Based Diabetic Retinopathy Screening
Aravind Eye Hospital in India integrated AI-driven screening for diabetic retinopathy to provide rapid, cost-effective diagnoses. The AI system analyzes retinal images from a large patient population, prioritizing high-risk cases for ophthalmologists. This implementation has significantly reduced diagnostic delays and improved access to early treatment for diabetic retinopathy, particularly in underserved regions.
Key Benefits of AI in Ophthalmic Diagnostics
Integrating AI in ophthalmology has significantly improved disease detection, workflow optimization, and patient care. By leveraging AI-powered diagnostics, ophthalmologists can provide more precise, timely, and efficient treatments.
Increased Accuracy and Efficiency
AI lowers human mistakes and improves diagnosis accuracy. It uses deep learning models that improve over time. These models learn from large amounts of patient data, helping them find even small signs of diseases like diabetic retinopathy or glaucoma.
AI-driven systems eliminate variability in human diagnoses, ensuring that every scan is assessed with the same level of accuracy and consistency.
As these models improve, they give eye doctors important insights, which help them create treatment plans for each patient.
AI-powered diagnostic tools analyze thousands of images in minutes. They identify subtle abnormalities that humans might miss. These tools use advanced image recognition to compare new scans with large databases of known disease patterns, helping doctors make faster and more accurate diagnoses.
These diagnostic tools integrate AI with telemedicine platforms to allow remote screenings, guaranteeing that patients in underserved regions receive timely evaluations.
AI’s ability to process vast amounts of imaging data reduces the burden on ophthalmologists. It allows them to focus on complex cases while AI systems handle routine screenings efficiently.
Faster Screening and Improved Patient Care
AI reduces diagnostic turnaround time. This allows ophthalmologists to focus on treatment and personalized patient care.
By leveraging AI-powered automation, diagnostic workflows become more efficient, minimizing the time between image acquisition, analyzing, and result interpretation.
AI enables instant image processing and comparison against extensive medical databases. It allows ophthalmologists to detect subtle abnormalities more rapidly than traditional methods.
Patients receive quicker diagnoses, leading to timely interventions and better outcomes. AI-driven screening ensures that high-risk cases are identified faster. This allows for proactive treatment planning and reduces the risk of disease progression.
By prioritizing urgent cases through AI-assisted triage, healthcare providers can better allocate resources. This ensures those with severe conditions receive immediate attention while maintaining streamlined workflows for routine cases.
Enhanced Ophthalmology Management
AI optimizes workflow efficiency in clinics and hospitals by streamlining administrative and clinical processes. Doing this reduces the burden on healthcare providers.
By integrating AI-driven systems, ophthalmology practices can:
- Enhance decision-making
- Minimize delays
- Improve overall patient management
AI-powered automation assists with:
- Patient scheduling
- Medical record organization
- Diagnostic imaging analysis
This reduces administrative bottlenecks and ensures smoother operations. Additionally, AI enables predictive analytics that helps clinicians:
- Anticipate patient needs
- Optimize resource allocation
- Enhance workflow coordination across departments
AI tools assist in:
- Scheduling
- Triaging urgent cases
- Predicting disease progression
Automated scheduling systems help manage patient appointments. They reduce wait times and ensure that critical cases receive prompt attention.
AI-powered triage algorithms analyze patient symptoms and diagnostic data to prioritize urgent cases. This allows ophthalmologists to focus on those at the highest risk.
Additionally, predictive analytics tools assess disease progression over time. They enable proactive intervention and personalized treatment planning.
Challenges and Limitations of AI in Ophthalmology
AI has demonstrated remarkable potential in ophthalmic diagnostics. However, its widespread adoption is not without obstacles.
Despite its advantages, AI faces challenges in AI implementation.
Integration Issues
AI adoption requires upgrading existing healthcare infrastructure. Many ophthalmology clinics and hospitals may lack the necessary hardware, software, and network capabilities to support AI-driven diagnostics.
Integrating AI requires investment in cloud computing, high-speed data processing, and advanced imaging equipment, which can be expensive.
Additionally, interoperability challenges between AI systems and existing electronic health records (EHR) platforms can create further roadblocks. This requires customized solutions to ensure seamless data sharing and workflow integration.
Training and Trust
Healthcare professionals must trust AI-generated insights and be trained to use them. Building confidence in AI requires comprehensive training programs that familiarize clinicians with the following:
- AI-driven diagnostics
- Their limitations
- Their applications in patient care
To help practitioners understand how AI tools work and how to interpret their outputs, these programs should include:
- Hands-on training
- Workshops
- Real-world case studies
Future AI Trends in Ophthalmology
The future of AI in ophthalmic diagnostics includes advancements in predictive analytics, AI-assisted robotic surgery, and smart diagnostic imaging. As AI-driven innovations evolve, they are expected to:
- Enhance early disease detection
- Automate surgical procedures
- Improve accessibility to high-quality eye care worldwide
Emerging AI Trends
As AI continues to evolve, its influence on ophthalmology expands beyond diagnostics. Emerging technologies are shaping the future of eye care, offering new ways to enhance treatment precision and accessibility.
Predictive AI Models
AI forecasts disease progression by analyzing historical patient data, imaging results, and genetic factors, allowing for proactive interventions. By identifying risk patterns early, AI helps ophthalmologists:
- Customize treatment plans
- Implement lifestyle adjustments
- Recommend early therapies to slow or prevent vision loss
AI in Robotic Surgery
AI-powered robotic systems assist in delicate eye surgeries by enhancing precision, reducing human error, and enabling minimally invasive procedures. These advanced robotic platforms integrate real-time AI-driven imaging, allowing surgeons to perform complex microsurgeries with unparalleled accuracy.
AI-assisted robotic systems are particularly beneficial in retinal surgery and cataract removal. Extreme precision is required to minimize risks and improve patient recovery outcomes.
AI-Driven Teleophthalmology
Remote AI diagnostics expand access to quality eye care worldwide. AI-powered teleophthalmology solutions enable real-time analysis of retinal images and other ocular data, allowing for accurate remote assessments.
These systems leverage deep learning algorithms to identify early signs of diabetic retinopathy, glaucoma, and macular degeneration.
The Future of Ophthalmic Diagnostics With AI
The impact of AI on ophthalmic diagnostics is undeniable. From early disease detection to precise diagnoses, AI-driven diagnostic tools are transforming eye care. AI enhances ophthalmology management, improves patient outcomes, and optimizes clinic workflows.
Clinics and hospitals looking to integrate advanced diagnostic tools can turn to Nava Ophthalmic, a leading supplier of top-quality ophthalmic equipment that offers innovative solutions tailored to various clinical needs and budgets.
Explore Nava Ophthalmic products and elevate your practice today.
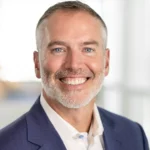
John Berdahl, MD
Meet Dr. Berdahl
Dr. Berdahl is most motivated by the trust his patients place in him during their moments of vulnerability.
That patient trust has, first and foremost, driven him to become an accomplished surgeon. However, as he meets patient needs and learns more about the problems they face, he’s had several opportunities to stretch his skills as an inventor and problem-solver. He co-invented the MKO melt, an innovation used in our Sioux Falls clinic that provides sedation during cataract surgery without the use of an IV or opioids, and developed Interfeen, a rare disease drug that helps with ocular conditions. He created astigmatismfix.com, a resource that has helped tens of thousands of surgeons eliminate residual astigmatism after cataract surgery, and he co-founded ExpertOpinion.MD, a site where patients can request medical opinions from authentic world experts. He also is the founder of Balance Ophthalmics, the first non-surgical, non-pharmacologic way to lower eye pressure for glaucoma treatment, which was FDA approved in 2024.
Interests & Added Expertise
Dr. Berdahl is equipped to employ the most innovative and tested techniques available to effectively treat most diseases of the anterior segment (front part of the eye). He is exceptionally skilled at diagnosing the best treatment for varying stages of glaucoma, corneal diseases, and cataracts. He is also a meticulous refractive surgeon.
To advance the technologies available to our patients, Dr. Berdahl collaborates with numerous ophthalmology companies as a consultant. However, to minimize potential bias, all consulting fees are donated to charity.
Education
- Hills-Beaver Creek High School, Hills, MN
- Augustana College, Sioux Falls, SD
- Mayo Medical School, Rochester, MN
- Mayo Clinic Internship, Scottsdale, AZ
- Duke University, Durham, NC
- Minnesota Eye Consultants Fellowship (Minneapolis, MN)